Early diagnostics of
Alzheimer’s Disease (AD)
Vitamin-AI’s goal is to improve the early diagnostics of Alzheimer’s Disease (AD)
among adults 45+ by affordable and AI-empowered mobile applications using
digital biomarkers.
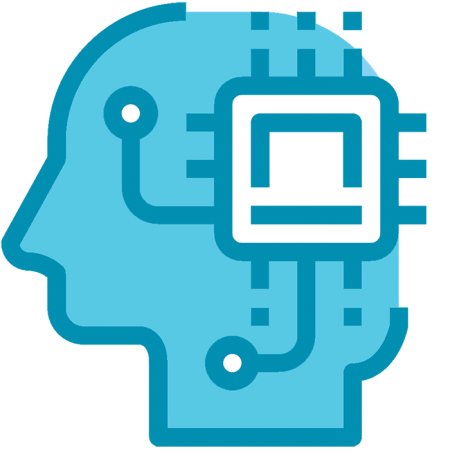
Digital biomarkers as alternative to physical interventions
There is a growing interest in innovative diagnostic tools for early AD detection which focuses on a wide variety of screening tools, including neuropsychometric measurements.
The recent literature univocally emphasizes the importance of technological and methodological innovation within the context of the rapid growth of Big Data and Artificial Intelligence. For this reason, we employ a strategy which combines state of the art methodological advances (including our own expertise and patented AI innovations) with high-quality data from different sources (voice, eye, psychometric).
Even if the predictive performance of the models is poor or moderate during the initial stages, we are confident that continuous improvements can be achieved as the amount of data keeps growing and our machine learning models are systematically updated.
Eye tracking and Alzheimer detection
Eye tracking has been used in early dementia prediction and has proven valuable in detecting AD. There are also studies that use other aspects of the eye to use as biomarkers such as pupil responses. Even though there are not many studies of early AD detection based on eye measurements, there seems to be sufficient reason to conclude that their use, in combination with other markers, is beneficial. In our experience, the predictive power of statistical models and Artificial Intelligence often depends on the presence of combined features from various origins.
Voice analysis and Alzheimer detection
Voice recordings have been successfully used in early detection of Parkinson’s Disease. Likewise, an early sign of dementia can be spotted in speech irregularities. Large volumes of data allow Machine Learning models to analyse the voice recordings and detect patterns or changes. Different aspects of the voice can show different information, but the general consensus is that speech patterns and irregularities are the most accurate way to predict abnormalities. As with the eye tracking methods, these tests work best when used in conjunction with other methods of Alzheimer’s detection.
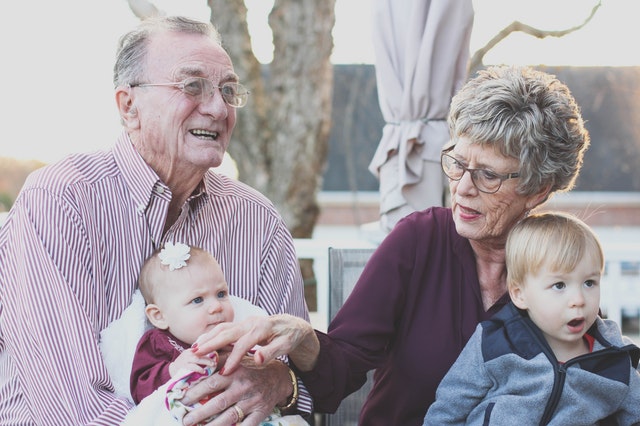
Alzheimer Disease
Vitamin AI will be a disruptive technology, which will contribute to the diagnostics of AD and improve the well-being of its patients.
Vitamin AI will have a major socio-economical impact. Economically it will reduce the medical and aged care costs and low income and productivity costs.
According to The Alzheimer Association (US) a person with AD costs yearly three times more than a person without AD. The last 5 years of the life of an AD patient costs 185.000 EUR versus 113.000 EUR for a heart patient and 112.000 EUR for a cancer patient.
Direct costs
Medical and aged care costs – We estimate that, if an early affordable diagnosis is provided, this will impact the quality of life for the patient. There will be no need of expensive medical interventions, medications, spending on caregivers and hospital stays (according to a study, dementia and related diseases cause a reduced life expectancy). The general practitioners will be able to monitor the brain and physical health of their patients remotely, thus have more time to focus on customized therapies and accurate diagnosis, as current diagnosis have a margin of error of 25%.
Indirect costs
Low income and productivity costs – By having better brain and health monitoring combined with an adequate health program, the productivity of the patient will improve, therefore he or she will be able to stay employed longer. On a social security side, there will be more benefit and less expense. Another impact will be the reduction of the burden that is upon relatives, friends and caregivers of AD patients. With our app, they can use the data to stay engaged and supportive in the recovery programs.
Main challenges
1
AD is diagnosed too late and prevention is little to none at that stage.
2
There is still no found cure for
Alzheimer Disease
3
Only 50% of the patients with cognitive impairments and AD are diagnosed.
4
There are about 25% faulty diagnoses within cognitive analysis.
5
The diagnoses are expensive and un-healthy (radioactive).
6
High costs for treatment to
society & families
AD is one of the top 10 deadliest diseases
Patients in an advanced stage of AD lose their memory, coordination and fall often. It is expected the number of dementia patients to be increasing as the population grows in both size and age – from 75 million to 132 million in 2050. The cost globally is 512 billion EUR, which is equivalent to 1% of the entire world’s gross domestic product.
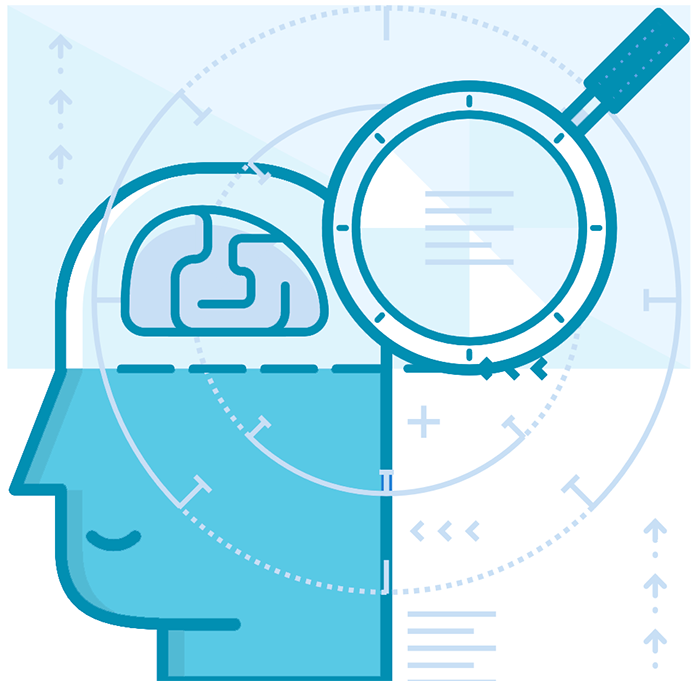
The healthcare AI market and AD
According to marketandmarkets.com research, AI in healthcare is expected to grow tenfold in the next 5 years or from 564 million EUR in 2016 to 6,7 billion EUR by 2022. Considering the fact that the population grows in both size and age, AI in healthcare is more than welcome as it will save costs and could potentially solve some of the big pains of our healthcare systems.
The estimated costs from AD worldwide were 692 billion EUR and they are estimated to more than double in 2030 to 1,69 trillion EUR. Over the past years there has been a considerable increase in the investments and focus for Alzhemer’s Disease research.
Artificial Intelligence / Machine Learning approach
From a methodological point of view there is a series of models that need to be considered. The following table provides an overview of some of the models that we have identified and describes the purpose, advantages, and risks in a non technical manner.
Vitamin AI will apply a stacked ensemble method. Our engine will develop several ML models and combine their use.
- Several time series models (varimax, arima, transfer function, recursive NN) used for feature engineering on recordings of eye tracking & voice. AA registered a specific patent on time series.
- Structural equation modeling with composite factors (PLS)
- Gradient Boosting, Random Forests, GLMs etc are used to optimize predictive performance
- End-to-end deep learning (and NN) will help in evaluating & improving the feature set
- Higher performance thanks to stacked ensemble methodology
- As alternative (and to challenge the above) we will use a Bayesian model (through MCMC/Gibbs sampling) which allows experts input.
From the data collection point of view we are relying on the collaboration of third parties which have agreed to provide their assistance. Patient data and data from a large social network of senior citizens should allow us to build a large database (5000+ individuals). Typical studies on AD detection are based on small samples which implies that it is impossible to employ Machine Learning, Artificial Intelligence, and other advanced statistical techniques.
Team & Scientific Board
Automated Analytics (further AA) was founded by Prof. Dr. Patrick Wessa (KU Leuven), MSc. Tom De Bast and MSc. James Troch for an artificial intelligence and machine learning engine that can be used universally for sectors such as med / health- / bio- and fine-tech. Our moto is ‘in data we trust ‘. AA primarily tries to detect patterns in volumes of data that can no longer be interpreted by humans. Prof. Dr. Patrick Wessa is an expert in artificial intelligence, machine learning, time series and responsible for AI/ML research at AA.
Every organization has large volume of data, but not all are capable to obtain insights from it. Some do not even know they can extract valuable information for further strategic decisions. Even if the organization is able to extract the data it usually takes too much time and too much effort. AA works on the foundation of data and builds intelligent software solution on the basis of artificial intelligence and learning, so companies can enable the power of their data, can gain access to it and leverage from it.
We realize AI powered solutions in some verticals like med/fin tech. In this Vitamin AI project, we collaborate with medical experts to use the power of data and realize a proof-of-concept in which we use digital biomarkers and an AI engine to do very early (15-20 year in advance) detection of Alzheimer’s disease. The innovation is to achieve this without expensive and radioactive scan, blood collection or puncture.
Management Team
-
Dr. Msc Msc Msc Hendrik Schwagten (PhD) Chief Medical Officer
Anti Aging Expert
-
Dr. Salza Palpurina (Phd)
AI expert
-
Marie Debrabandere (Msc)
Neurodegenerative brain disease expert
Advisors
-
Mary Lou Ackerman (Msc) Advisor
VP innovation Saint Elizabeth (Canada)
-
Dr. Ivan Popov (PhD) Expert
Senior Data Scientist Vitamin AI
Molecular biologist/Bioinformatician
-
Apr. Annemiek Van Hese (MsC) Advisor
Anti Aging Expert
-
Dr. Rishi Panday (Phd) Advisor
Neurology & Clinical Neurophysiology
Road Map
We are currently looking for test users. If you are interested, please sign up as beta user for our Vitamin-AI app.
Contact Us
SEND US A MESSAGE
CE Certified
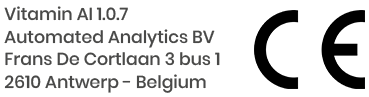